Preamble
In this post, we ran 2 experiments with 10 random seeds across 2000 iterations in the Swimmer Gather domain. We used the code from Rein Houthooft's VIME-TRPO algorithm for the csv files associated with the vime_1, ..., vime_10 dataframes. We then modified the "intrinsic reward" function using an analogous function from financial risk theory called Entropic Value at Risk (EVaR) to generate the csv files associated with the mime_1, ..., mime_10 dataframes.Note that 5 graphs are presented. The first graph shows each of the 10 randomly seeded executions of the VIME-TRPO algorithm. Note that almost half of the trajectories fail to get above 0.3 which represents 30% of the available reward. The second graph shows the average of all 10 randomly seeded executions of the VIME-TRPO algorithm. The performance is shown to be between 0.3 and 0.4.
The third graph shows each of the 10 randomly seeded executions of our modified VIME-TRPO algorithm. Note that none of the trajectories are below 0.3 in performance after 500 iterations. The fourth graph then shows that the average performance of all 10 randomly seeded executions of the modified VIME-TRPO algorithm is between 0.6 and 0.7.
The fifth graph shows the average results of the VIME-TRPO algorithm in blue and the average results of our modified VIME-TRPO algorithm in orange. This constitutes a near doubling of average peformance, but it must be emphasized that this is mainly due to improved robustness of the learning algorithm due to the use of EVaR.
Import Experiment Data
import numpy as np
import matplotlib
import pandas as pd
mime_1 = pd.read_csv ('trpo-expl_2018_12_15_08_50_56_0001/progress.csv')
mime_2 = pd.read_csv ('trpo-expl_2018_12_15_08_50_56_0002/progress.csv')
mime_3 = pd.read_csv ('trpo-expl_2018_12_15_08_50_56_0003/progress.csv')
mime_4 = pd.read_csv ('trpo-expl_2018_12_15_08_50_56_0004/progress.csv')
mime_5 = pd.read_csv ('trpo-expl_2018_12_15_08_50_56_0005/progress.csv')
mime_6 = pd.read_csv ('trpo-expl_2018_12_15_08_50_56_0006/progress.csv')
mime_7 = pd.read_csv ('trpo-expl_2018_12_15_08_50_56_0007/progress.csv')
mime_8 = pd.read_csv ('trpo-expl_2018_12_15_08_50_56_0008/progress.csv')
mime_9 = pd.read_csv ('trpo-expl_2018_12_15_08_50_56_0009/progress.csv')
mime_10 = pd.read_csv ('trpo-expl_2018_12_15_08_50_56_0010/progress.csv')
vime_1 = pd.read_csv ('./trpo-expl_2019_01_22_12_56_49_0001/progress.csv')
vime_2 = pd.read_csv ('./trpo-expl_2019_01_22_12_56_49_0002/progress.csv')
vime_3 = pd.read_csv ('./trpo-expl_2019_01_22_12_56_49_0003/progress.csv')
vime_4 = pd.read_csv ('./trpo-expl_2019_01_22_12_56_49_0004/progress.csv')
vime_5 = pd.read_csv ('./trpo-expl_2019_01_22_12_56_49_0005/progress.csv')
vime_6 = pd.read_csv ('./trpo-expl_2019_01_22_12_56_49_0006/progress.csv')
vime_7 = pd.read_csv ('./trpo-expl_2019_01_22_12_56_49_0007/progress.csv')
vime_8 = pd.read_csv ('./trpo-expl_2019_01_22_12_56_49_0008/progress.csv')
vime_9 = pd.read_csv ('./trpo-expl_2019_01_22_12_56_49_0009/progress.csv')
vime_10 = pd.read_csv ('./trpo-expl_2019_01_22_12_56_49_0010/progress.csv')
List of Fields
Which outputs the variable names:print(0,vime_1.columns[0])
print(1,vime_1.columns[1])
print(2,vime_1.columns[2])
print(3,vime_1.columns[3])
print(4,vime_1.columns[4])
print(5,vime_1.columns[5])
print(6,vime_1.columns[6])
print(7,vime_1.columns[7])
print(8,vime_1.columns[8])
print(9,vime_1.columns[9])
print(10,vime_1.columns[10])
print(11,vime_1.columns[11])
print(12,vime_1.columns[12])
print(13,vime_1.columns[13])
print(14,vime_1.columns[14])
print(15,vime_1.columns[15])
print(16,vime_1.columns[16])
print(17,vime_1.columns[17])
print(18,vime_1.columns[18])
print(19,vime_1.columns[19])
Will use StdReturn (normalized reward) for performance comparison.
0 MaxReturn
1 LossAfter
2 BNN_DynModelSqLossAfter
3 BNN_DynModelSqLossBefore
4 AverageReturn
5 Expl_MaxKL
6 Iteration
7 AverageDiscountedReturn
8 MinReturn
9 Expl_MinKL
10 dLoss
11 Entropy
12 AveragePolicyStd
13 StdReturn
14 Perplexity
15 MeanKL
16 ExplainedVariance
17 Expl_MeanKL
18 NumTrajs
19 Expl_StdKL
VIME Plots
import matplotlib.pyplot as plt
vime_1[vime_1.columns[13]].plot('line')
plt.show
vime_2[vime_2.columns[13]].plot('line')
plt.show
vime_3[vime_3.columns[13]].plot('line')
plt.show
vime_4[vime_4.columns[13]].plot('line')
plt.show
vime_5[vime_5.columns[13]].plot('line')
plt.show
vime_6[vime_6.columns[13]].plot('line')
plt.show
vime_7[vime_7.columns[13]].plot('line')
plt.show
vime_8[vime_8.columns[13]].plot('line')
plt.show
vime_9[vime_9.columns[13]].plot('line')
plt.show
vime_10[vime_10.columns[13]].plot('line')
plt.show
<function matplotlib.pyplot.show(*args, **kw)>
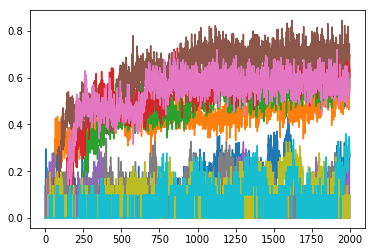
VIME Average Plot
vime_11 = (vime_1[vime_1.columns[13]]+vime_2[vime_2.columns[13]]+vime_3[vime_3.columns[13]]+vime_4[vime_4.columns[13]]+vime_5[vime_5.columns[13]]+vime_6[vime_6.columns[13]]+vime_7[vime_7.columns[13]]+vime_8[vime_8.columns[13]]+vime_9[vime_9.columns[13]]+vime_10[vime_10.columns[13]])/10
vime_11.plot('line')
plt.show
<function matplotlib.pyplot.show(*args, **kw)>
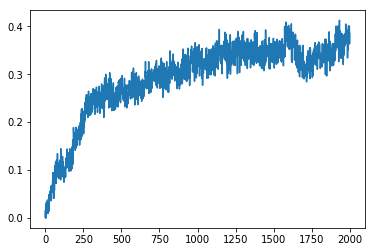
VIME Star Plots
import matplotlib.pyplot as plt
mime_1[mime_1.columns[13]].plot('line')
plt.show
mime_2[mime_2.columns[13]].plot('line')
plt.show
mime_3[mime_3.columns[13]].plot('line')
plt.show
mime_4[mime_4.columns[13]].plot('line')
plt.show
mime_5[mime_5.columns[13]].plot('line')
plt.show
mime_6[mime_6.columns[13]].plot('line')
plt.show
mime_7[mime_7.columns[13]].plot('line')
plt.show
mime_8[mime_8.columns[13]].plot('line')
plt.show
mime_9[mime_9.columns[13]].plot('line')
plt.show
mime_10[mime_10.columns[13]].plot('line')
plt.show
<function matplotlib.pyplot.show(*args, **kw)>
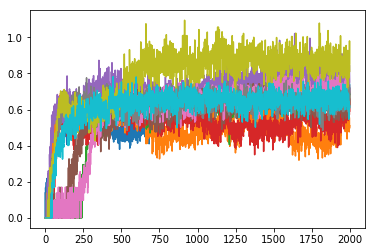
VIME Star Average Plot
mime_11 = (mime_1[mime_1.columns[13]]+mime_2[mime_2.columns[13]]+mime_3[mime_3.columns[13]]+mime_4[mime_4.columns[13]]+mime_5[mime_5.columns[13]]+mime_6[mime_6.columns[13]]+mime_7[mime_7.columns[13]]+mime_8[mime_8.columns[13]]+mime_9[mime_9.columns[13]]+mime_10[mime_10.columns[13]])/10
mime_11.plot('line')
plt.show
<function matplotlib.pyplot.show(*args, **kw)>
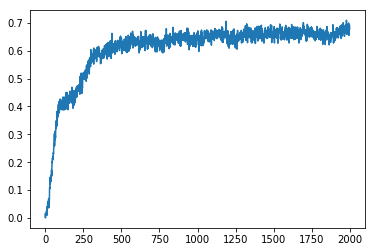
VIME and VIME Star Average Plots on Same Figure
vime_11 = (vime_1[vime_1.columns[13]]+vime_2[vime_2.columns[13]]+vime_3[vime_3.columns[13]]+vime_4[vime_4.columns[13]]+vime_5[vime_5.columns[13]]+vime_6[vime_6.columns[13]]+vime_7[vime_7.columns[13]]+vime_8[vime_8.columns[13]]+vime_9[vime_9.columns[13]]+vime_10[vime_10.columns[13]])/10
vime_11.plot('line')
plt.show
mime_11 = (mime_1[mime_1.columns[13]]+mime_2[mime_2.columns[13]]+mime_3[mime_3.columns[13]]+mime_4[mime_4.columns[13]]+mime_5[mime_5.columns[13]]+mime_6[mime_6.columns[13]]+mime_7[mime_7.columns[13]]+mime_8[mime_8.columns[13]]+mime_9[mime_9.columns[13]]+mime_10[mime_10.columns[13]])/10
mime_11.plot('line')
plt.show
<function matplotlib.pyplot.show(*args, **kw)>
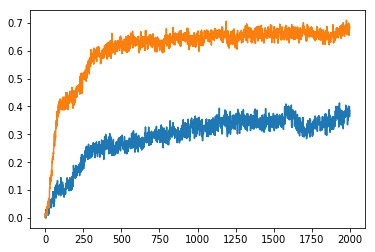